(Much of the material adapted from notes from Easterbrook and Neves)
Research Checklist
- Pick a topic
- Identify the research question(s)
- Check the literature
- Identify your philosophical stance
- Identify appropriate theories
- Choose the method(s)
- Design the study
- Unit of analysis?
- Target population?
- Sampling technique?
- Data collection techniques?
- Metrics for key variables?
- Handle confounding factors
- Critically appraise the design for threats to validity
- Get IRB approval
- Informed consent?
- Benefits outweigh risks?
- Recruit subjects / field sites
- Conduct the study
- Analyze the data
- Write up the results and publish them
- Iterate
What is a Randomized Controlled Experiment (RCT)?
…experimental investigation of a testable hypothesis, in which conditions are set up to isolate the variables of interest (“independent variables”) and test how they affect certain measurable outcomes (the “dependent variables”)
What is an RCT Good for?
- Quantitative analysis of benefits of a particular tool/technique
- Establishing cause-and-effect in a controlled setting
Limitations:
- Difficult to apply if you cannot simulate the right conditions in the lab
- Limited confidence that the laboratory setup reflects real situations
- Ignores contextual factors (e.g. social/organizational/political factors)
- Extremely time-consuming!
RCTs are generally used to determine causal effects
Causality
- Does X cause Y?
- Does X prevent Y?
- What causes X?
- What effect does X have on Y?
Causality-Comparative
- Does X cause more Y than does Z?
- Is X better at preventing Y than is Z?
- Does X cause more Y than does Z under one condition but not others?
Some RCT Vocab:
Independent Variables: Variables (factors) that are manipulated to measure their effect. Typically select specific levels of each variable to test
Dependent Variables: “output” variables – tested to see how the independent variables affect them
Treatments. Each combination of values of the independent variables is a treatment
Simplest design: 1 independent variable x 2 levels = 2 treatments; e.g. tool A vs. tool B
Subjects: Human participants who perform some task to which the treatments are applied. Note: subjects must be assigned to treatments randomly.
Hypothesis Testing:
Start with a clear hypothesis, drawn from an explicit theory
This guides all steps of the design:
- E.g. Which variables to study, which to ignore
- E.g. How to measure them
- E.g. Who the subjects should be
- E.g. What the task should be
Set up the experiment to (attempt to) refute the theory:
$𝐻_0$: the null hypothesis – “the theory does not apply”. Usually expressed as no effect – the independent variable(s) will not cause a difference between the treatments
$𝐻_0$ assumed to be true unless the data says otherwise.
$𝐻_1$: the alternative hypothesis – “the theory predicts…”
If $𝐻_0$ is rejected, that is evidence that the alternative hypothesis is correct
Assigning Treatments to Subjects
Between Subjects Design:
Different subjects get different treatments (assigned randomly)
- Reduces load on each individual subject
- Increases risk that confounding factors affect results
- E.g. differences might be caused by subjects varying skill levels, experience, etc
- Handled through blocking: group subjects into “equivalent” blocks
- Note: blocking only works if you can identify and measure the relevant confounding factors
Within Subjects Design:
Each subject tries all treatments
- Reduces chance that inter-subject differences impact the results
- Increases risk of learning effects
- E.g. if subjects get better from one treatment to the next
- Handled through balancing: vary order of the treatments
- Note: balancing only works if learning effects are symmetric
Multiple Factors Design
Crossed Design
- Used when factors are independent
- Randomly assign subjects to each cell in the table
- Balance numbers in each cell!
- E.g. 2×2 factorial design:
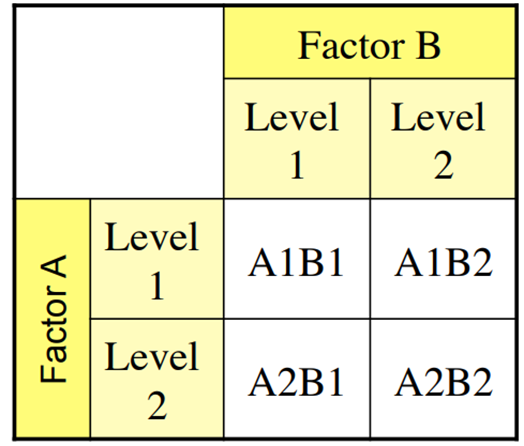
Nested Design
- Used when one factor depends on the level of the another
- E.g. Factor A is the technique,
- Factor B is expert vs. novice in that technique
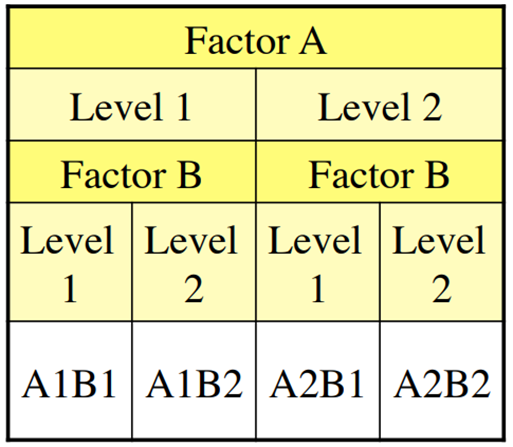
Detecting Effects

Lavrakas, P.J., 2008. Encyclopedia of survey research methods. Sage publications
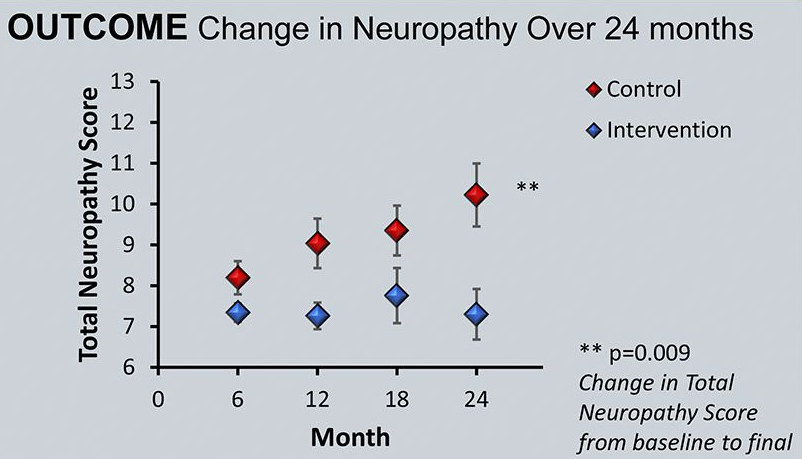
Arnold, R., Pianta, T.J., Pussell, B.A., Kirby, A., O’Brien, K., Sullivan, K., Holyday, M., Cormack, C., Kiernan, M.C. and Krishnan, A.V., 2017. Randomized, controlled trial of the effect of dietary potassium restriction on nerve function in CKD. Clinical Journal of the American Society of Nephrology, 12(10), pp.1569-1577.
When do we not use RCTs?
- When you can’t control the variables
- When there are many more variables than data points
- When you cannot separate phenomena from context
- Phenomena that don’t occur in a lab setting
E.g. large scale, complex software projects - Effects can be wide-ranging.
- Effects can take a long time to appear (weeks, months, years!)
- When the context is important
E.g. When you need to know how context affects the phenomena - When you need to know whether your theory applies to a specific real world setting
Positivist View of RCT Validity
Construct Validity
Are we measuring the construct we intended to measure?
Did we translate these constructs correctly into observable measures?
Did the metrics we use have suitable discriminatory power?
Internal Validity
Do the results really follow from the data?
Have we properly eliminated any confounding variables?
External Validity
Are the findings generalizable beyond the immediate study?
Do the results support the claims of generalizability?
Empirical Reliability
If the study was repeated, would we get the same results?
Did we eliminate all researcher biases?