My research lies at the intersection of machine learning, network science and social media. Generally speaking, I am interested in uncovering how humans consume and curate information.
The Weninger Lab has worked on several funded projects over the past many years.

Projects
Advancing Media Literacy for New Digital Arrivals in Developing Countries
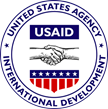
Lead PI – Chief of Party
2018–2025
Source: USAID
At a time when communities around the world increasingly turn to digital source for information, online and social media systems play a critical role in affecting attitudes and behavior. A core problem is that social media channels are being manipulated by malicious groups to spread misinformation in low and middle income countries (LMIC) to exacerbate social divides and influence citizen involvement in democratic processes. The spread and adoption of misinformation through digital channels is especially problematic because many users of online and social media systems are not aware of how (mis)information is spread through these channels. Notre Dame’s goal is to improve media literacy in low and middle income countries (LMIC) especially among new digital arrivals through a targeted digital media literacy campaign. Specifically, the team research question is: if Notre dame can provide customized online media literacy content to segmented audiences of new digital arrivals, then the recipients of media literacy will be less likely to engage with and spread misinformation.
EDIFICE: Early Detection of Influence Indicators with Machine Intelligence
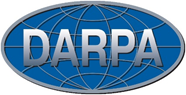
Notre Dame Lead PI – Sub to USC/ISI
2021–2025
Source: INCAS – Defense Advanced Research Projects Agency (DARPA)
The goal of this project is to create a cognitive agent simulation framework for studying social behavior in online information environments. We are developing a scalable, virtual laboratory, calibrated on real-world data, for studying dynamics of online social phenomena and information diffusion at different temporal resolutions and at multiple scales, from individual to community to global collective behavior.
Individual agent models within COSINE will be based on first-principles of human behavior uncovered through empirical analysis of the vast troves of online behavioral data. These models will incorporate bounded rationality and cognitive biases within models of attention. In addition, COSINE’s multi-resolution, scalable framework will enable time-resolved, massive simulations of dynamic information environments.
DISCOVER: A Data-Driven Integrated Approach for Semantic Inconsistencies Verification
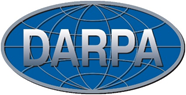
Notre Dame co-PI with Walter Scheirer and sub to Purdue
2021–2024
Source: Semafor- Defense Advanced Research Projects Agency (DARPA)
Different from traditional digital forensics techniques –– which often focus on determining whether or not a digital object is fake –– this research project aims at looking at a series of digital objects from different modalities as a population in a specific context to be studied. We aim at leveraging contextual associations to pinpoint essential clues about a chain of events leading to a particular observed fact. The context might be vital to understanding the semantics behind the objects and also the truth behind the story, empowering valuable fact-checking sets of solutions
to fight fake news, misinformation, and political propaganda.
CAREER – Principled Structure Discovery for Network Analysis
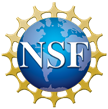
Lead PI
2017–2022
Source: National Science Foundation
The discovery and analysis of network patterns is central to the scientific enterprise. Thus, extracting the useful and interesting building blocks of a network is critical to the advancement of many scientific fields. Indeed the most pivotal moments in the development of a scientific field are centered on discoveries about the structure of some phenomena. For example, chemists have found that many chemical interactions are the result of the underlying structural properties of interactions between elements. Biologists have agreed that tree structures are useful when organizing the evolutionary history of life, sociologists find that triadic closure underlies community development, and neuroscientists have found “small world” dynamics within neurons in the brain. In other instances, the structural organization of the entities may resemble a ring, a clique, a star, a constellation, or any number of complex configurations. Unfortunately, current graph mining research deals with small pre-defined patterns or frequently reoccurring patterns, even though interesting and useful information may be hidden in unknown and non-frequent patterns. Principled strategies for extracting these complex patterns are needed to discover the precise mechanisms that govern network structure and growth. This is exactly the focus of this project: to develop and evaluate techniques that learn the building blocks of real world networks that, in aggregate, succinctly describe the observed interactions expressed in the network.
Mining Conversation Trails for Effective Group Behavior
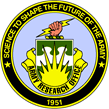
Lead PI
2017–2021
Source: Army Research Office
Our key contributions lie in the treatment of human conversation and discussion as trails over a concept graph. With this perspective, an individual’s ideas (as expressed through language) can be mapped to explicit entities or concepts, and a single argument or train-of-thought can be treated as a trail/walk over the nodes in a concept graph. Therefore, within a group discussion we will first translate the stated positions, arguments, and stories into a set of distinct paths over a concept graph. Once in graph form, group dynamics can be analyzed as a new type of graph mining problem where agents synchronously traverse concepts, and where existing graph mining methods can be applied to answer many new, interesting questions about the nature of human discussion.
Unlike existing network models where nodes represent individuals and information flows over the edges between the individuals, our key idea is to flip this model such that network nodes represent concepts over which individuals walk during a group discussion. In this new mode of thinking important scientific questions will be addressed — although we expect to raise more questions than we answer. For example: How do we map group discussion to a concept graph? Do conversation trails match human paths through existing knowledge graphs? What, if any, graph patterns exist in the conversation trails? How can we leverage outcomes from previous discussions to predict aspects of leadership, social cognition, and group decision making? The study of conversational trails for effective group behavior is a critical step towards a deeper understanding of the complex interactions between and among individuals.
COSINE: Cognitive Online Simulation of Information Network Environments
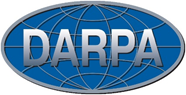
Notre Dame Lead PI – Sub to USC/ISI
2017–2021
Source: SocialSim – Defense Advanced Research Projects Agency (DARPA)
The goal of this project is to create a cognitive agent simulation framework for studying social behavior in online information environments. We are developing a scalable, virtual laboratory, calibrated on real-world data, for studying dynamics of online social phenomena and information diffusion at different temporal resolutions and at multiple scales, from individual to community to global collective behavior.
Individual agent models within COSINE will be based on first-principles of human behavior uncovered through empirical analysis of the vast troves of online behavioral data. These models will incorporate bounded rationality and cognitive biases within models of attention. In addition, COSINE’s multi-resolution, scalable framework will enable time-resolved, massive simulations of dynamic information environments.
Deception Detection, Tracking and Factuality Assessment in Social and News Media
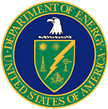
Lead PI
2017–2019
Source: Department of Energy
Deception Detection and Tracking LDRD Project with Pacific Northwest National Laboratory (PNNL) and University of Notre Dame will (Task 1) analyze evolution of deceptive news content on Reddit, and (Task 2) study deceptive video propagation and influence on YouTube. The focus tasks will involve running quantitative analysis and machine learning experiments to measure deception propagation and evolution in social networks. Such research will add to the foundation of social network analysis and machine learning used by academic, government and industrial agencies to measure information propagation and evolution in social media.
Socio-Digital Influence Attack Models and Deterrence
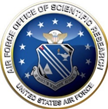
Lead PI
2014–2017
Source: Air Force Office of Scientific Research
We rely on ratings contributed by others to make decisions about which hotels, books, movies, political candidates, news, comments, and stories are worth our time and money. The sheer volume of new information being produced and consumed only increases the reliance that individuals place on anonymous others to curate and sort massive amounts of information. Given the widespread use and economic value of socio-digital voting systems, it is important to consider whether they can successfully harness the wisdom of crowd to accurately aggregate individual information. There is a giant gap in our knowledge and capabilities in this area, including untested and contradictory social theories. Fortunately, these gaps can be filled using new experimental methodologies on large, socio-digital data sets. We will be able to determine if these socio-digital platforms produce useful, unbiased, aggregate outcomes, or (more likely) if, and how, opinion and behavior is influenced and manipulated. Work of our own and recent tangential experiments suggest that decisions and opinions can be significantly influenced by minor manipulations yielding different social behavior.
Based on these early indications, we will:
(1) Determine the manner by which social influence affects decision making and opinion formation in online social spaces; and
(2) Determine the causal relationship among influences, i.e., the extent to which aggregate opinion affects aggregate rating, and the extent to which ratings affect aggregate opinion.
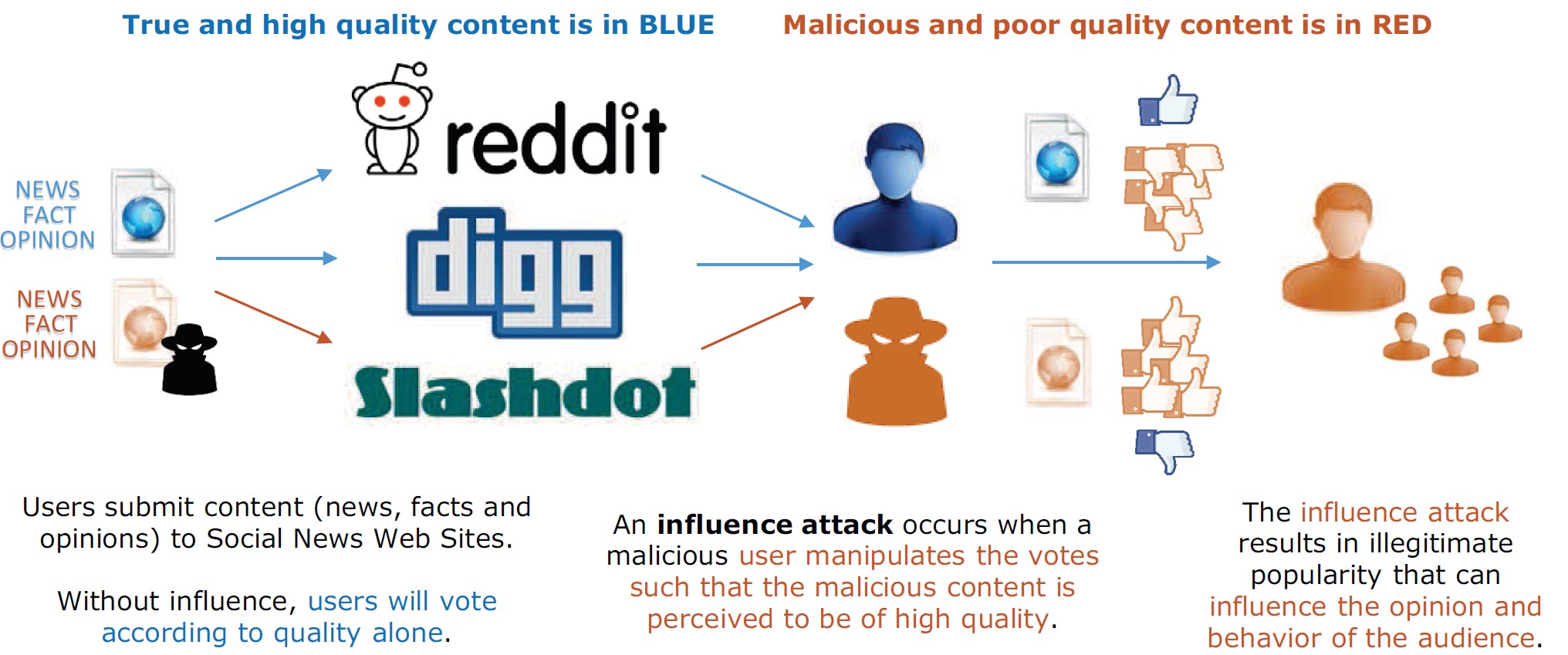
These determinations will be made via a set of experiments on various social news aggregation and commentary Web sites (hereafter referred to as social news sites) like Slashdot, Digg and Reddit. Based on statistical analysis of the experimental data we will be able to make conclusions regarding the manner by which social influence in online social spaces affects general decision making and opinion formation, and we will use these conclusions to:
(3) Formulate a general model of influence attacks in social media.
The results of these objectives are of critical interest to military intelligence and Military Information Support Operations (MISO, generally known as Psychological Operations (PsyOps)). Conflicts on the modern battlefield are successful not only with tanks and guns, but by winning hearts and minds. This is certainly not a secret; reports from recent conflicts have uncovered politically motivated computer hackers targeting American and Western digital assets. For example, the Syrian Electronic Army (SEA) – a collection of pro-government computer hackers aligned with Syrian President Bashar al-Assad – has successfully, although temporarily, crashed and/or defaced dozens of American Web sites.11 Foreign PsyOps groups are actively manipulating votes and ratings in social media platforms, and may therefore be actively manipulating Western public opinion. Based on these developments we will:
(4) Use the results and models developed from objectives 1–3 to develop deterrence strategies against foreign or malicious influence attacks may have on user behavior.
Knowledge Hierarchies and Natural Navigation
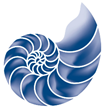
Notre Dame Lead PI – Sub to University of Chicago
2017–2019
Source: Templeton Foundation
Open information extraction (OIE) extracts and learns relationships from free text; it is the data gathering process used to create the most widely-used knowledge networks. Extractable facts and relationships will vary greatly within the corpora, but in general we could expect to find support for declarations, assertions, theories and results, e.g., protein x interacts with protein y (describing protein protein interaction), or Facebook group x reacts to Facebook group y (describing information cascades), among countless other possibilities. The result these extractions will be a large set of “triples” describing the objects and the relationship, with links back to the source document, as well as the support (normalized count) of the fact, which is an indication of the triples’ accuracy and/or veracity.
This data would then be made available to the Metaknowledge group and the scientific community at large. The extraction technology is rather straightforward, and there would be very little “research risk” involved, but admittedly the scientific novelty is also low. However, the significance and possible impact could be very large because no one has ever attempted OIE on a large scientific corpus before. The resulting dataset would be seed downstream novel and impactful downstream research and likely be used by many other members of the Metaknowledge network and the wider research community just as dbpedia, freebase and other knowledge networks have seeded many excellent downstream tasks.